Ag Tech Talk Podcast: Bringing Artificial Intelligence and Humans Together to Solve Edge Cases
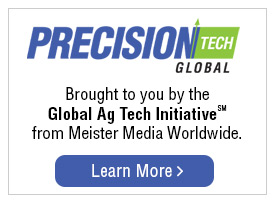
It’s 2023. According to past predictions we were supposed to have flying cars taking us to the school and office, jetpacks that allowed us to visit the store without getting into traffic jams, menial task would be handled by robots, and artificial intelligence would either solve the world’s problems or enslaving mankind. While technically, those things do exist, they’re far from ubiquitous and a far cry from the promise of decades ago. Existing artificial intelligence and automation are pretty sophisticated but can get quickly stumped by unexpected “edge cases.” SparkAI’s solution brings the human factor together with existing technology to overcome those unique challenges. In this podcast we talk with Michael Kohen, Founder and CEO of SparkAI about artificial intelligence and the future of farming.
Transcript of the podcast:
AgriBusiness Global: Why don’t you tell us what SparkAI is? Give me your elevator speech about what that’s all about?
Michael Kohen: So, look, here’s the specific problem we solve. And it really has to do with the reality of the world that we live in the real world is unpredictable and complex. And as a result, it’s really hard to build AI systems that work perfectly always.
We come from the self-driving car industry. You wouldn’t get into a self-driving car that was 95% accurate. So, for a lot of applications, and especially ones that are dealing with either things related to safety or productivity and output it’s that last 5% that is, the critical gap that’s preventing these next generation applications from getting to market.
And it’s there because again, AI systems work really, really well, 95%t of the time. But when they encounter something that they don’t quite understand things start to break down, and that impacts the viability of the product. So that’s the piece that we solve. We do that by leveraging technology and methodologies that we originally built when we were working on self-driving cars.
MK: We do it in a particular, unique way by combining our own AI systems with input from real human beings. And we do that in real time. The result is that it helps our customers cross a variety of applications, launch and scale AI products faster than they ever thought possible. And before their AI systems are perfect.
ABG: Forgive my ignorance, here. We have artificial intelligence. We’ve been hearing about that for a long time, and then automation, robotics, that kind of thing which is kind of what you’re marrying here. If I understand you explain how those are different, and how they complement each other.
MK: Sure. When you’re talking about robotics, typically what you’re referring to is the physical, mechanical – machines, right? Whether they be autonomous, mobile robots, an autonomous tractor, or picking robots or goods to personal robots like ones that might be employed at a at a warehouse. What you’re talking about are physical things that are interacting with the world in a physical way.
Those robots, and especially the next generation of those robots, are leveraging AI, which itself is a fairly broad umbrella concept. But think of it as the body and the brain. So, the robot is the body. The AI system is the brain.
You need both of those in order to really deliver products that deliver on the value that they’re trying to deliver. Automation is another one of these fairly broad umbrella terms that depending on how you want to define probably encapsulates AI and robotics into this concept of automation. That’s how the distinction lines up.
ABG: Okay. So, you mentioned automobiles. We’ve been hearing about self-driving autos, but we also hear about accidents and things like that, and I think that’s kind of what you referenced when you mentioned the 5%. On your website you call them edge cases. Can you talk a little bit more about what those are?
MK: Like a busy, busy city like San Francisco City. You are constantly observing the environment and adapting to the some of the crazy things that you sometimes might be encountering for the first time. Again, we humans really get to take advantage of the decades of life experience that we have, but also millions of years of human evolution that equip us incredibly well to adapt to changing situations in the world very quickly, based on mental models that again leverage our past life, experience and our past evolutionary experience. That concept just doesn’t exist for AI, today. The way we build AI is, we have some base model, and we pummel it with what’s called training data – as many examples, if you will, of particular things that we expect that model to encounter. But there’s only so much of that data available in the world, and there’s only so much of that that an AI system can confidently ingest and really start building a mental model around. So, what happens is most AI teams are able to do that really, really well and get to the performance of let’s, say 95% or 96%. The challenge is that last 5% represents what are called long-tail edge cases or long-tail exceptions. What’s referring to is all those situations, the long tail of situations that might exist in the world that you can’t predict and you cannot train for, and you can’t really build a big repository of training data because they are rare.
They’re rare on an individual basis, but in aggregate they represent a meaningful percentage of the problems.
So again, 95% of the time, your self-driving car might be working perfectly. But in that you know that there’s 5% of the time when the world looks slightly different. If that system can’t adapt, you know you’ve got a real dangerous situation in your hands.
ABG: Your solution is to bring that human factor back into automation. Can you talk a little bit about how that works? If a grower has his sprayer out there, whatever tool he’s using on the field. If he can do it with artificial intelligence, you can do it in the middle of the night, right? How does bringing humans back into this really work. Does that sort of defeat the purpose of relying on artificial intelligence?
MK: Not so much. I mean, we really view what we’re doing here as a cornerstone of the AI revolution. What I mean by that is, this is going to take a few decades to get these AI systems to perform perfectly well on their own always. This is a story that unfolds genuinely over decades. When you think about the applications in agriculture? Specifically, growers have been depending on a particular way of doing things for centuries. And now you have new technology that’s coming in. And sure, if it works well most of the time, great. But if you turn whatever machine you have running in the middle of the night, and it does something wrong and destroys your field or impacts you in some negative way you really can’t rely on that product anymore.
It’s really not about dampening our ability to launch and scale AI products. It’s really more about, how do we take the systems that we, as an industry, have built today and give growers the confidence that these systems will run extremely well and reliably all the time, and part of that is helping AI system bridge this this cognitive gap that today only humans have. It gives people the confidence to say, this is something that I can rely on to really do the thing that I’m handing off to do.
ABG: Okay. So, maybe now is a good time for a specific example. Give me something in that 4% to 5% that that artificial intelligence isn’t going to be able to figure out on its own.
MK: Sure. We’re fairly public about our relationship with John Deere, which is just an incredible, incredible company. Look at some of the headlines that are coming out around some of the work they’ve been doing over the past number of years to really introduce automation into the solutions that they deliver for their end customers. It’s really one of the most important automation companies in existence today that is really delivering on the promise of automation and delivering on the value there.
One of the use cases that we’ve been working closely with them on is this autonomous tractor. Here you have a system that involves a fairly large piece of equipment that’s out there navigating the world on its own. As many of the folks listening in on this on this podcast know, a farm field is actually a fairly unstructured environment – unpredictable and potentially complex. Every so often you have a shopping cart in the middle of the in the of the field. And you need to be able to handle that safely and confidently in a way that doesn’t impact performance. The way we interface with a product like that is in those moments where the system might encounter something that it’s not quite sure what it is.
Instead of just stopping and waiting for the farmer to come and help, it’ll call our system automatically. We’ll get data from the robots. We’ll see kind of through the eyes of the robot and provide real human beings trained for the use case. Look at that data and are able to provide a cognitive common sense human input. That input goes directly back to the robot, which then uses it to make a safe and confident decision in the field.
Again, the robot can make a safe and confident decision and everything I just talked about happens in a matter of seconds so really seamless in terms of the functioning of the robot itself.
ABG: Obviously the more products you sell and the more times you at this SparkAI solution is implemented out in the field you’re going to need more and more people available to answer these issues.
MK: This is where the technology layer comes in. The brute force way is just hire this massive sea of people that are always available to respond within seconds. But the real magic of what we’ve developed here – and again it’s built out of the tech that we built in self-driving cars – is the ability to do more with less and have a smaller human footprint that doesn’t need to scale linearly with the number of deployments that we might have with a customer or customers and help enable the humans to do a faster, more accurate job through technology. There’re jobs and routing systems – real-time decision tools that enable humans to do a faster job. All that comes together to really deliver that same job with as few people as possible.
ABG: Okay, speaking of a few people as possible, one of the regular complaints or concerns we hear from growers is about labor issues, trying to find people on the farm. An automated or artificial intelligence solution would seem to be a possible mitigating circumstances, so that that issue is a little less concerning. But are there other things that may be preventing people from adopting this kind of technology.
MK: There is increasing pressure on growers to produce, right? And that pressure is coming from a lot of different directions all at once – increasing population that’s more developed and consuming more, pressure from climate, and soil conditions from weather, and from labor, shortages in labor, and the ability to really meet supply and demand. So, all that pressure is really fueling this interest and desire to think about how to solve parts of that puzzle with automation.
The interest is there. The question, then, becomes, how reliable is that automation today? We humans are incredibly forgiving of other humans making errors or making mistakes. But as soon as you take a supposedly intelligent AI system, and say, ‘Hey, you should trust this, that AI system really degrades your confidence in that in that product, and ability for you to really trust it. So, I think there’re a few dynamics, right? One is that that are potentially impacting adoption of robotics, and I am in the field. One is that overall performance and reliability factor. Another is some of those applications haven’t really been perfected yet – from just purely a mechanical standpoint getting articulating robots to pick every single strawberry in a row. It’s actually a particularly hard physics challenge. And the third kind of interesting piece. And I think this is just a unique factor of agriculture is, yes, some people go into agriculture because they want to be outdoors, and you know they want to work with their hands, and they want to be part of the act of growing. So. finding that right balance of how to enable people to continue to do that while enabling them to answer this call and pressure from the need to be more and more productive.
You know it’s a balance that I think we as an industry are still trying to find together.
ABG: You mentioned you work with John Deere. How does it physically work? Is it a software solution that’s embedded? Is there a box that’s sitting, on the dashboard that works within. What’re the mechanics that actually says, ‘Here’s the thing that is that has the artificial intelligence embedded in it.’
MK: Yeah, so this is the beautiful part. We really interface at the decision layer. So, there’s no physical hardware that we’re introducing. We’ve talked about packages into what’s called an API, which is just a way for two software systems to communicate with each other. So, with all of our customers. They have a robotic system. That system has onboard Intelligence, and it knows when it’s not confident in a decision. So, when it knows that it’s not confident it’ll call our system automatically, a software-to-software interface and then we do our thing in the background and then deliver a response. It’s all via software, so that allows us to interface with a variety of different types of robotic systems and customers.
We work with a variety of applications within agriculture, but also outside of agriculture – insurance, tech, logistics, warehouse, drone inspection. Those are all areas that we spend a lot of time in.
ABG: One thing that we’ve heard a lot about recently is data – companies collecting data. You’re collecting scenarios to learn so that you can help the artificial intelligence continue to learn. Are you collecting other types of data on farm data? And if so how do you make sure that you’re not giving away any information that a grower doesn’t want to be out there.
MK: Yeah. We’re there to help our customers deliver a better product to their to their customers. In terms of that data relationship that’s managed between our customer and their end customer. We don’t own any of that data. We’re really there just to service our own customers. So really we leave that to them to manage, and we’re just a supporter of that.
ABG: We’ve been hearing about technology and how it’s going to change the world. Maybe I watched “The Jetsons” cartoon way too many times as a child. We’re supposed to have flying cars by now. The promise of these solutions seems to outpace the reality of them. You mentioned it might take decades. Where are we going to be five years from now? Are you still going to have that at a human connection to your artificial intelligence or is it going to be completely automated?
MK: The reality here is this is a story that plays out over decades. And I think, what the industry is starting to awaken to is this idea that AI is hard to perfect, and it’s hard to make reliable and work perfectly as I mentioned in every single possible scenario. So, what you’re hearing rom, the senior most AI practitioners and thought leaders out there is this increasing theme that the pace of development that we’re on is great. But there’s this other track that is, as of yet, undiscovered that we as an industry, need to figure out. But the running theme is that that other path needs to start looking a lot more like human cognition, right? Not that I’m saying human cognition needs to be part of this forever and ever, but that the way we build AI systems needs to start taking some more queues from how human cognitive systems work. And we need to start figuring out a way to build in that common-sense reasoning into how we build AI systems, which again is really a to be discovered track. In AI there is probably a Nobel prize or two in that story. It’s a once in a decade innovation that takes place.
ABG: Are we closer to a fully autonomous car or fully autonomous tractor?
MK: Fully autonomous tractor. And I think that’s one of the most beautiful things about agriculture where you’re going to start seeing a lot more large-scale autonomous systems and not just robots, but also just reliance on AI in a farm setting. You’re going to see that a lot sooner than in an urban environment. I firmly believe this, the AI revolution starts on the farm.
ABG: You mentioned a grocery cart in the middle of the field somewhere. Can you maybe give me a very specific example of or two of an edge case that that your system has had to solve.
MK: Sure. So, the way you can think about edge cases are really just exceptions. There’re two ways to think about it. Either it’s something completely unique that you could never have predicted, and is totally new to the system, or it’s something totally mundane. But for whatever reason, in that moment it looks unique. That’s maybe the easier one to think about a log in the middle of a field, or a boulder, or a or a bush on the edge of a field.
For whatever reason, there are times where that bush, because of how the sun is oriented or it’s a particularly dusty day. For whatever reason that combination of environmental factors makes that bush look like something completely different. For those that drive tesla’s and are brave enough to turn autopilot on, you’ll see these things happening every so often where a car is identified as a truck or a person is identified as a bicyclist. So that’s the same that happens really anywhere an AI system is deployed.
And the Ai system is intelligent enough to say, “Hey, this is weird, and I’m also not confident about. I’m not confident about how weird that is.”
A very specific example I can give you is just going to sound so mundane a bush at the edge of a at the edge of a field that looks that looks like something else again because of some environmental condition.
I truly do believe that the AI revolution starts on the farm, and there’s a tremendous amount of promise to meet a very specific need on a human level, helping feed the world. And there’s ways to do that through robotics.
And there’s ways to do this through some of the other really exciting AI systems that are being introduced over the past few years and into the future around, using AI to better manage crops – crop yield, pest detection, crop health, soil monitoring.
Some of those systems are fairly simple. They’re systems that sit in the field and just breathe the air and produce results. Others involve drones that are doing inspection on crops and trying to identify evidence of certain crop health issues. There’s a lot happening across agriculture. The linchpin of all of this is how reliable can you make it? How much can you get farmers to trust the output of these systems that are feeding all this data back? And then also, how do you package this data in a way that actually allows the farmer to take action.
If you know, one corner of the field is affected by fall army worm, how can the farmer take action on just that corner of the field, if you know their watering system is the entire row. So, there’s a lot of this kind of symbiosis that needs to happen. It starts with developing better, more reliable systems that really prove the ROI case to increase adoption. And then through that, there’s an ongoing evolutionary code development that happens over time.
ABG: We talked a little bit earlier about our focus on crop inputs. How do you see artificial intelligence tools affecting crop inputs?
MK: It comes down to optimization. And you see this both with robotic systems and non-robotic systems. Some of the running themes are: How do you? get more yield out of your crops with greater efficiency? That’s less fertilizer pesticides, optimizing water usage. These are things that are important to the downstream, the overall cost of managing a farm, increasing the output and yield of your farm, but also you to have implications to (ensure) a healthy climate and a healthy environment.
There’s a lot of work to be done there. The better that data becomes, the more digestible it’s presented to farmers, the more decisive actions that can be taken
ABG: How do they find out more about your offerings?
MK: They can find us at www.spark.ai. All the information is up there, and we talk a lot about various use cases in agriculture as well as a handful of thought pieces that really start to talk about what it’s going to take to introduce these next generation products to market. Not five years from now or 10 years from now, but right now, today, when the world needs it most.
ABG: Okay, thank you very much for your time. I really appreciate your insights today.
MK: Absolutely, really a pleasure to be here.